
Dr Sathiya Narayanan S
Assistant Professor, VIT-Chennai

Greedy Pursuits Assisted Basis Pursuit
Fusion based Compressive Sensing (CS) reconstruction algorithms combine multiple CS reconstruction algorithms, which worked with different principles, to obtain a better signal estimate. Modified CS algorithms such as Modified Basis Pursuit (Mod-BP) ensured a sparse signal can efficiently be reconstructed when a part of its support is known.
Since Mod-BP makes use of available signal knowledge to improve upon BP, we propose to employ multiple Greedy Pursuits (GPs) to derive a partial support for Mod-BP. As Mod-BP makes use of signal knowledge derived using GPs, we term our proposed algorithm as Greedy Pursuits Assisted Basis Pursuit (GPABP).
Modified Adaptive Basis Pursuits
In Distributed Compressive Sensing (DCS), correlated sparse signals stand for an ensemble of signals characterized by presenting a sparse correlation. If one signal is known apriori, the remaining signals in the ensemble can be reconstructed using l1-minimization with far fewer measurements compared to separate CS reconstruction. Reconstruction of such correlated signals is possible via Modified-CS and Regularized-Modified-BP. However, these methods are greatly influenced by the support set of the known signal that includes locations irrelevant to the target signal.
While recovering each signal, prior to Modified-CS or Regularized-Modified-BP, we propose an adaptation step to retain only the sparse locations significant to that signal. We call our proposed methods as Modified-Adaptive-BP and Regularized-Modified-Adaptive-BP. Theoretical guarantees and experimental results show that our proposed methods provide efficient recovery compared to that of the Modified-CS and its regularized version.
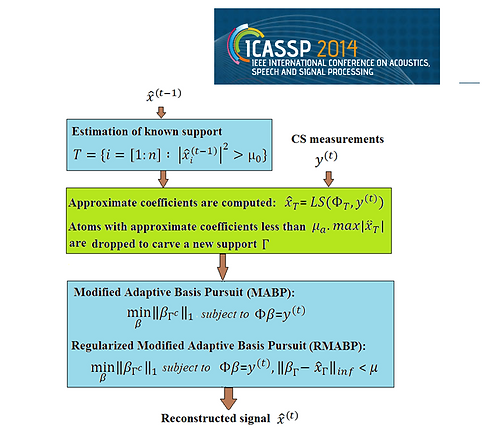